Adversarial Robustness in Neural Networks
Neural networks have demonstrated outstanding performance in recognition tasks. However, these methods are highly vulnerable to imperceptible corruptions in the input images.
Ground truth
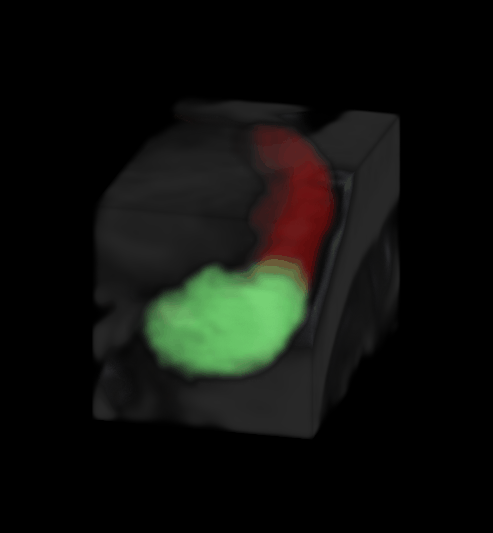
Prediction on a clean image
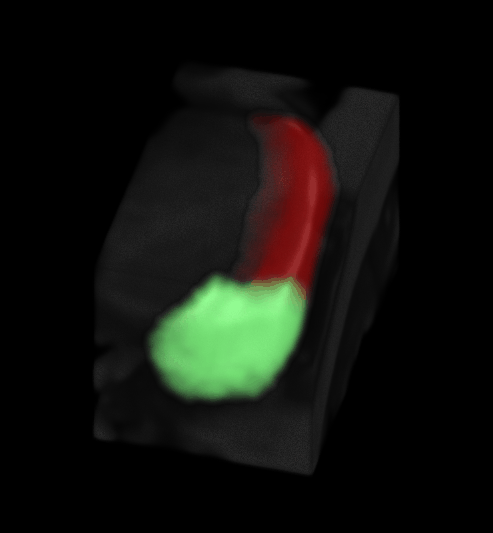
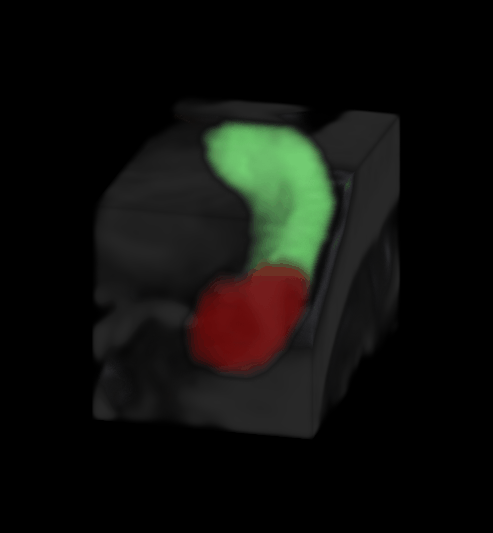
RObust General Segmentation (ROG)
We introduce a lattice architecture for general medical image segmentation
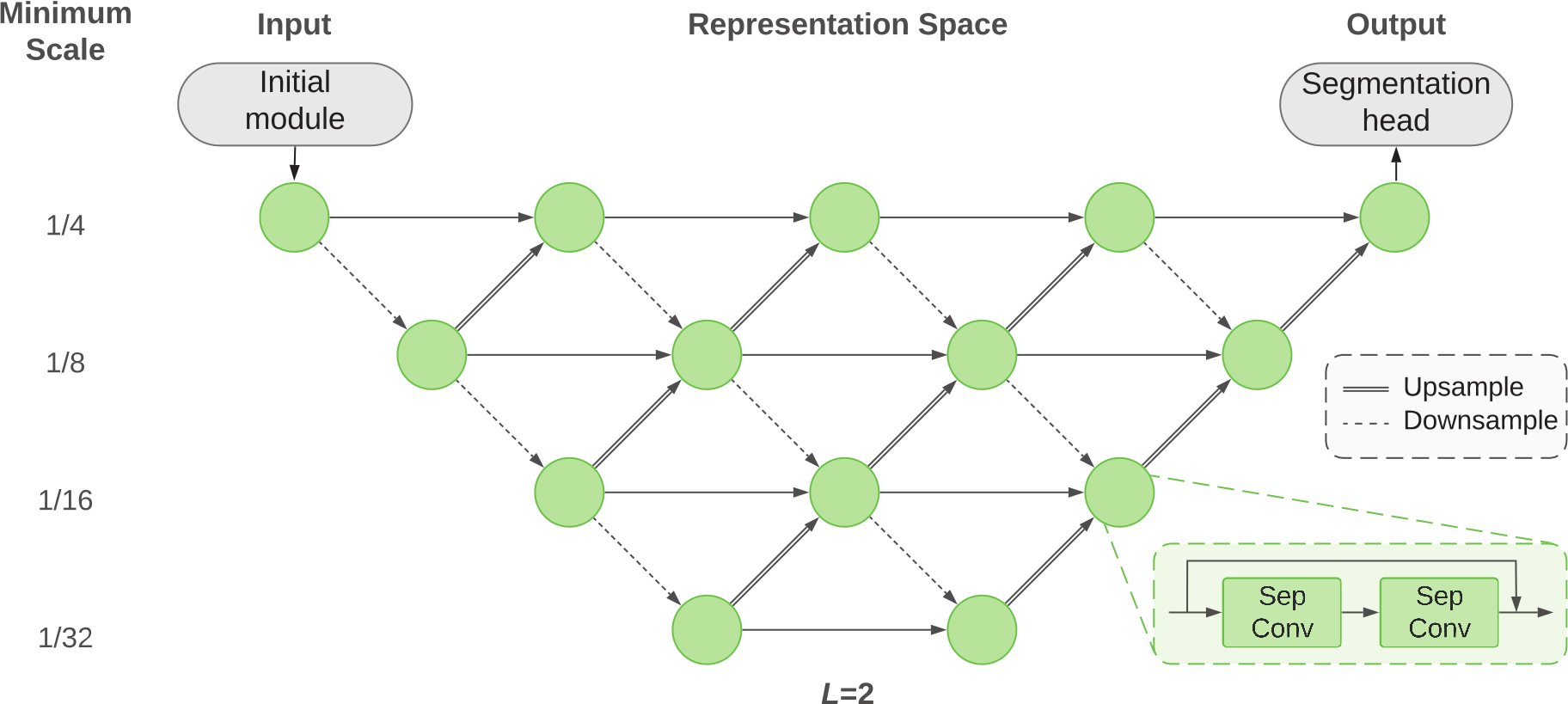
Adversarial Robusntess Benchmark
We introduce a new benchmark to reliably assess adversarial robustness on the Medical Segmentation Decathlon. Our benchmarck includes 5 state-of-the-art adversarial attacks that we expanded to the domain of 3D segmentation:
- PGD
- APGD-CE
- APGD-DLR (only for non-binary tasks)
- FAB
- Square Attack
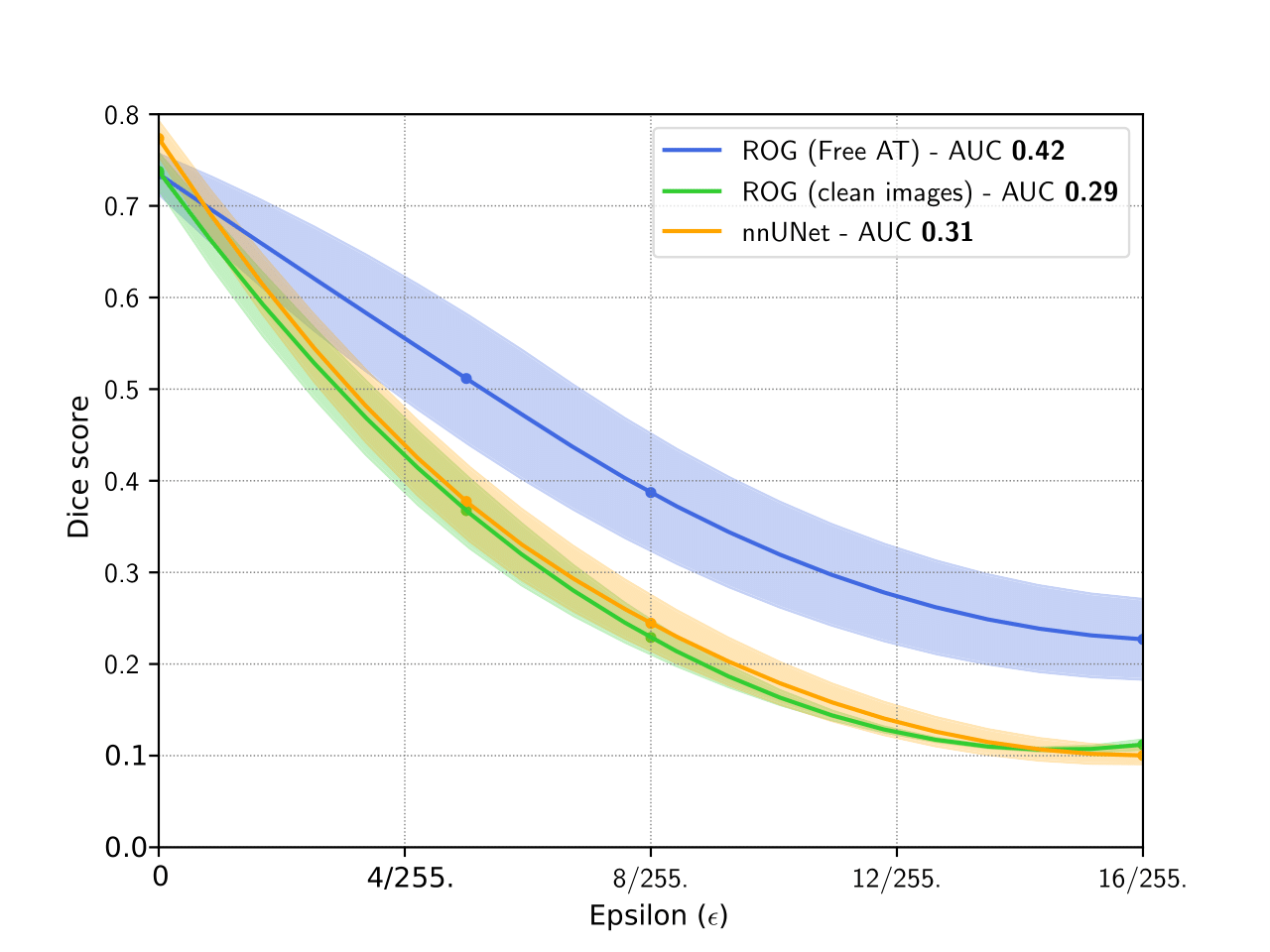
Paper
You can get access to our full paper here. If you find our work useful, please use the following BibTeX entry for citation:
@inproceedings{daza2021towards,
title={Towards Robust General Medical Image Segmentation},
author={Daza, Laura and P{\'e}rez, Juan C and Arbel{\'a}ez, Pablo},
booktitle={MICCAI},
year={2021}
}
Team
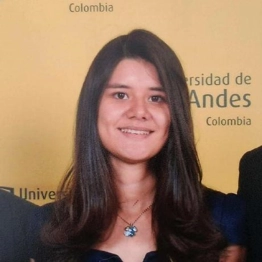
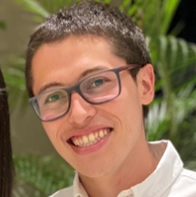
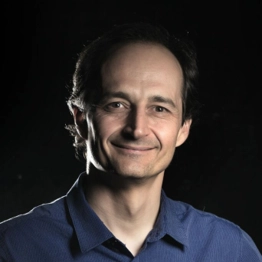